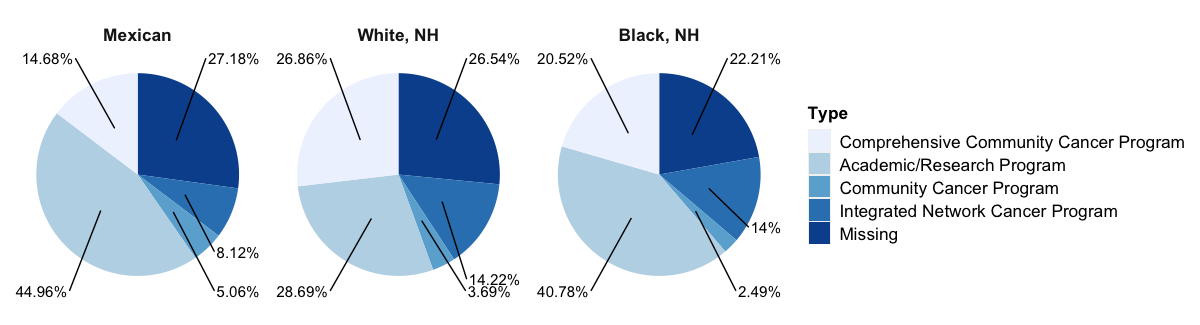
In this tutorial, I will demonstrate how to create a pie chart using the ggplot2
and ggrepel
packages in R. A pie chart is a type of chart that displays numerical proportions of a variable in polar coordinates, similar to a bar chart. However, unlike a bar chart, a pie chart focuses on displaying percentages rather than raw counts. To create the chart, I will use the publicly available National Cancer Database (NCDB) to display the percentage of combinations of race and ethnicity with the type of cancer facility.
1. Dataset
After data cleaning, the dataset I used to create pie charts includes four variables:
-
Type
: this is a categorical variable that is used to indicate the type of cancer clinic [5 levels: ‘Academic/Research Program’, ‘Community Cancer Program’, ‘Comprehensive Community Cancer Program’, ‘Integrated Network Cancer Program’, ‘Missing’] (string)
-
Origin
: this is a categorical variable that is used to indicate race/ethnicity group [3 levels: ‘Black, NH’, ‘White, NH’, ‘Mexican’] (string)
-
Count
: this is a numerical variable that is used to indicate a count for patients in the combination of Type
and Origin
(numerical)
-
Percentage
: this is a numerical variable that is used to indicate a percentage for patients in the combination of Type
and Origin
(numerical)
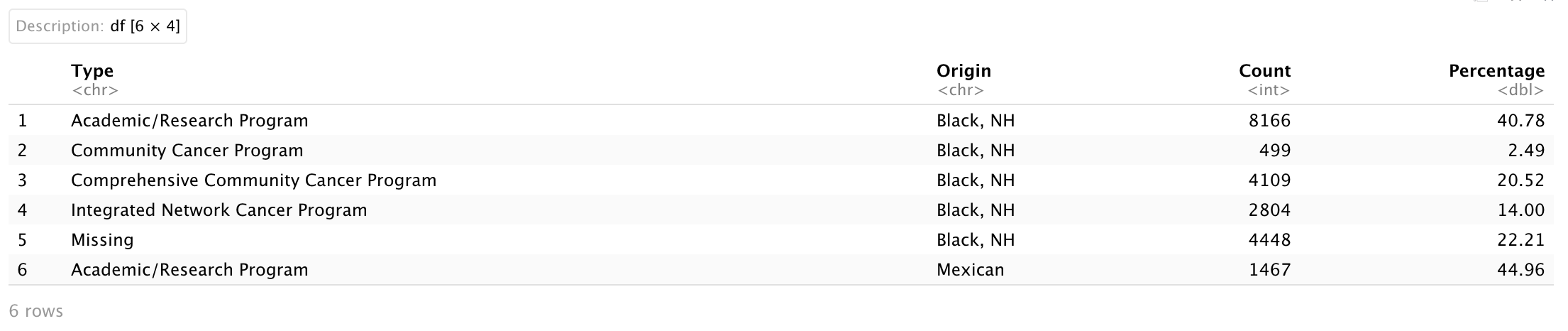
I will use this simple dataset to first create a single pie chart for Mexican
patients, and then multiple pie charts for the other patients. First, I will create a variable to display percentage labels in a pie chart. I will use the paste0()
function to concatenate the numerical value of the Percentage
with a percentage sign (%), and store the result as a string variable. And then I will set Origin
and Type
as factors.
# Percentage label
Dt.Plot$'Percentage.Label' <- paste0(Dt.Plot$Percentage, '%')
# Factor for Origin and Type
Dt.Plot$Origin <- factor(Dt.Plot$Origin, levels = c("Mexican", 'White, NH', 'Black, NH'))
Dt.Plot$Type <- factor(Dt.Plot$Type, levels = c("Comprehensive Community Cancer Program",
"Academic/Research Program",
"Community Cancer Program",
"Integrated Network Cancer Program",
"Missing"))
In R, a pie chart maps a bar chart into polar coordinates. If a user wants to label percentages in a pie chart, they must provide coordinates for Percentage.Label
in the polar system. I am going to use arrange()
, desc()
, group_by()
, and mutate()
functions from the dplyr
package and cumsum()
function in R base.
Here, I arranged the dataset by Origin
and sorted it in decreasing order based on the values in Type
.
-
group_by()
: it takes a data frame and one or more variables to group by
-
mutate()
: it creates new columns that are functions of existing variables
-
cumsum()
: it returns a vector whose elements are the cumulative sums
An original bar plot from geom_bar()
or geom_col()
stacks rectangles for stratified variables vertically, and I plan to place the Percentage.Label
in the middle of each rectangle.
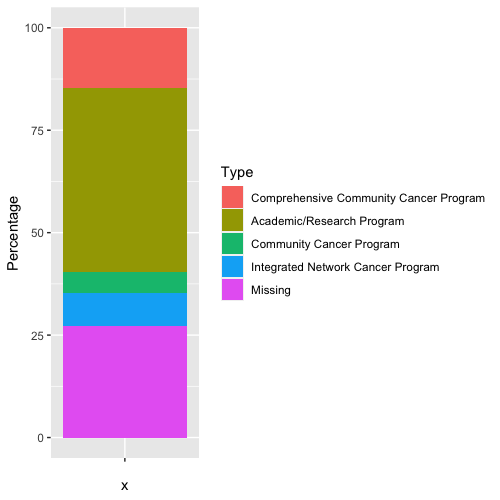
This can be done by first grouping the data frame by the Origin
variable, and then subtracting half of the Percentage
from the cumulative sum of Percentage
for each Origin
.
# Pie chart
Dt.PieChart <-
Dt.Plot %>%
arrange(Origin, desc(Type)) %>% # arrange it with Origin and decreasing order of Type
group_by(Origin) %>% # group by Origin
mutate(text_y = cumsum(Percentage) - Percentage/2) # the middle of each rectangle
head(Dt.PieChart)
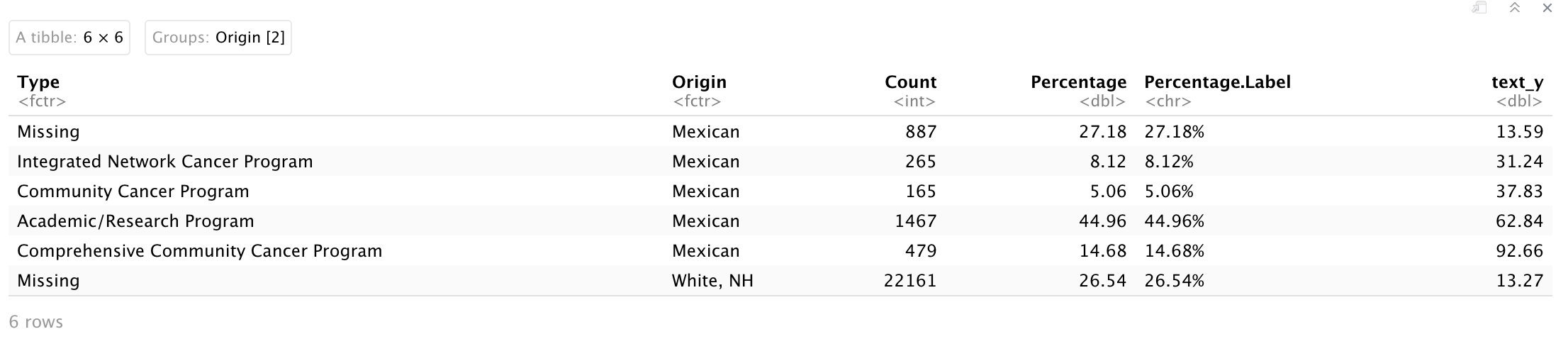
Dt.PieChart
will be used to create pie charts!
2. Single pie
To create a single pie chart, three functions are needed to be used:
-
geom_col()
: it uses the heights of the bars to represent values in the data (equivalent to stat = 'identity'
in geom_bar()
)
width
: it controls the bar width
-
coord_polar()
: it transforms a stacked bar chart into polar coordinates
theta
: it is an argument that map angle to axes (x or y)
-
geom_text_repel()
: it is from the ggrepel
package and adds text directly to the plot and I will use some arguments below:
-
size
: it controls the size of text/label
-
show.legend
: it controls if we want to show legend of text/label
-
nudge_x
or nudge_y
: it is an argument to perform horizontal and vertical adjustments to nudge the starting position of each text label.
I will extract a subset of Dt.PieChart
for 'Mexican'
and create a pie chart to show the percentage of facility type for Mexican patients.
Dt.Mexican <- Dt.PieChart[which(Dt.PieChart$Origin == 'Mexican'), ]
ggplot(Dt.Mexican,
aes(x = '', # We don't want to show x axis
y = Percentage, # y axis is numerical percentage
fill = Type)) + # color for each facility type
geom_col(width = 1) + # create a bar chart with width = 1
coord_polar(theta = "y") + # transfer y axis into polar coordinate
scale_fill_brewer() + # color
geom_text_repel(aes(y = text_y,
label = Percentage.Label), # label text
size = 4, # size of text
show.legend = FALSE, # remove legend
nudge_x = 1.5) + # the distance between text and figure
theme(axis.text = element_blank(),
axis.ticks = element_blank(),
axis.title = element_blank(),
panel.grid = element_blank(),
panel.background = element_blank(),
plot.background = element_blank(),
legend.background = element_blank(),
legend.title = element_text(size = 13, face = 'bold'),
legend.text = element_text(size = 13),
strip.text = element_text(size = 13, face = 'bold'),
strip.background = element_blank())
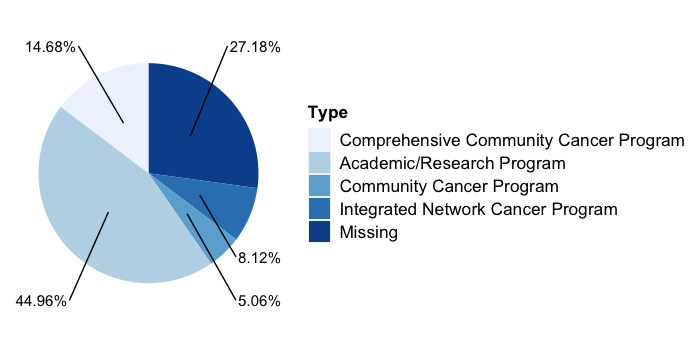
3. Multiple pies
For multiple pie charts, we will need to use facet_grid()
functions to form a matrix of panels defined by row and column faceting variables.
ggplot(Dt.PieChart,
aes(x = '', # We don't want to show x axis
y = Percentage, # y axis is numerical percentage
fill = Type)) + # color for each facility type
geom_col(width = 1) + # create a bar chart with width = 1
coord_polar(theta = "y") + # transfer y axis into polar coordinate
scale_fill_brewer() + # color
geom_text_repel(aes(y = text_y,
label = Percentage.Label), # label text
size = 4, # size of text
show.legend = FALSE, # remove legend
nudge_x = 1.5) + # the distance between text and figure
facet_grid(cols = vars(Origin), # faceted by Origin variable
scales = 'fixed') +
theme(axis.text = element_blank(),
axis.ticks = element_blank(),
axis.title = element_blank(),
panel.grid = element_blank(),
panel.background = element_blank(),
plot.background = element_blank(),
legend.background = element_blank(),
legend.title = element_text(size = 13, face = 'bold'),
legend.text = element_text(size = 13),
strip.text = element_text(size = 13, face = 'bold'),
strip.background = element_blank())
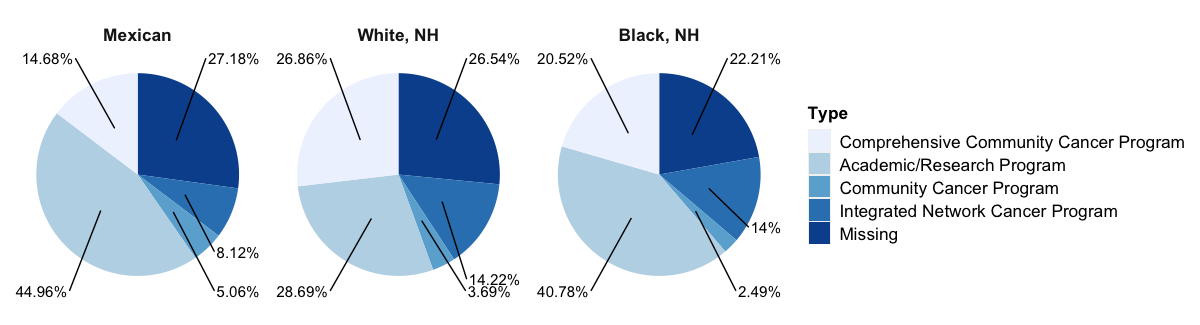
Here we go!